Project in Progress
Large-scale Brain Network Modeling

Mapping directed information flows along the cortical hierarchy

Younghyun Oh 오영현
My research utilizes neuroimaging data, dynamical systems theory and modeling techniques, and AI methods (especially recurrent neural networks) with the primary aim of understanding how the signal is processed across the entire brain. Ongoing projects include, i) in-vivo cartography of state-dependent signal flow hierarchy in the human cerebral cortex, ii) interoceptive AI framework for autonomous and adaptive artificial agents, iii) whole-brain effective connectivity of the sensorimotor system using 7T fMRI with electrical microstimulation in non-human primates, and iv) intrinsic effective connectivity as the basis of functional localization in the cortex.

Generative modeling of functional brain network development
This study investigates age-related changes in thalamocortical connectivity and their role in functional network specialization during early development. Infancy connectivity favors sensorimotor networks, guided by genetic factors, while childhood sees expansion to the salience network and differentiation of external and internal systems. Simulations support thalamic influence on brain maturation, emphasizing its critical role during adolescence and implications for cognitive disorders.

Elucidating the role of hippocampal-neocortical connectivity and replay in continual learning

Hyunhoe An 안현회
How does the brain learn new tasks by leveraging prior knowledge? My research explores the neural mechanisms that enable efficient integration of new information while preserving existing knowledge. Specifically, I focus on hippocampal-cortical connectivity through the lens of the complementary learning systems theory, studying how the hippocampus enables rapid learning and how the cortex consolidates information for long-term generalization. I also investigate the role of traveling waves—patterns of neural activity that facilitate communication between these brain regions—to balance plasticity and stability in continual learning. Understanding these dynamics could provide insights into how the brain generalizes knowledge across different tasks and environments.
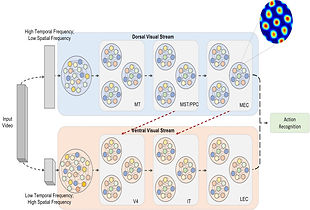
A unified computational mechanism in the dorsal stream for motion

Hyewon Kang 강혜원
A Unified Computational Mechanism in the Dorsal Stream for Motion Perception and Compositional Generalization : This study presents a computational model of the dorsal visual stream, emphasizing its role in motion perception and visual generalization. Unlike previous models that focus on static processing via the ventral stream, our approach highlights the dorsal stream’s dynamic nature—characterized by high temporal frequency processing, fast conduction (magnocellular input), and input to grid cells in the medial entorhinal cortex (MEC). The model incorporates biologically inspired inductive biases, including short-term plasticity, lateral interactions, selective attention, orientation columns, and recurrent connectivity. By modeling these dorsal-specific properties, we offer a unified mechanism for motion perception and compositional generalization.

Life-span profiling of whole-brain substrates for continual learning

Jungmin Lee 이정민

Yebin Park 박예빈
Continual learning is fundamental to human intelligence, enabling adaptation and knowledge transfer across different tasks throughout life. This study investigates the neural basis of lifelong learning by examining key brain substrates—modularity/compositionality, cortical hierarchy, neurogenesis, neural subspace and memory reactivation—using resting-state, task, and movie fMRI, as well as anatomical MRI. By modeling these features across the lifespan, we aim to reveal how the brain maintains general intelligence from development to aging.
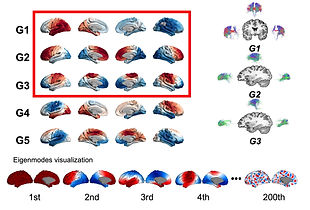
Organizational principles of structural brain network in the primate species

Sunghyung Hong 홍성형
Structural connectivity (SC) from diffusion tractography captures whole-brain connections but faces challenges due to high dimensionality. This study applied a low-dimensional mapping method to create structural gradients (SG) representing connectivity smoothly across the brain. It also separately analyzed short- and long-range connectivity impacts on SG patterns and explored how cortical geometry influences these gradients, aiming to identify key geometric constraints on brain structure.
Representation of neuronal information across the distributed systems
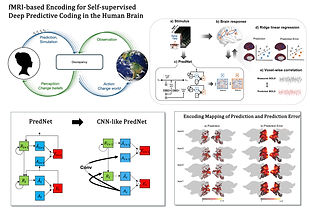
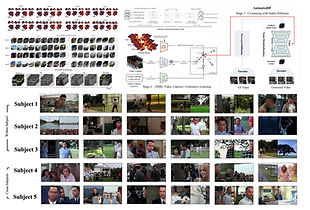
In-vivo mapping of predictive coding in the human visual cortices
Brain encoding-decoding during movie watching based on generative AI

Jungmin Lee 이정민

Myeonggyo Jeong 정명교
Anticipating future outcomes is key to human cognition, enabling adaptive responses to dynamic environments. Predictive coding theory describes the brain as a self-supervised hierarchical generative model, minimizing prediction errors by integrating top-down and bottom-up inputs. Despite its significance, whole-brain empirical evidence remains limited. To address this, we applied PredNet (a convLSTM model) to analyze temporal activation dynamics in movie-watching fMRI data, using voxel-wise encoding to examine predictive processes and error representations across the brain.
My research explores brain decoding by reconstructing visual stimuli from functional magnetic resonance imaging (fMRI) BOLD signals. Using movie-watching fMRI data, I employ generative AI models, including Stable Diffusion and multimodal transformers, to decode and synthesize dynamic visual representations. In addition to decoding, I conduct encoding analyses to examine how different model components account for neural activity, providing insights into the computational principles underlying visual perception.This work aims to deepen our understanding of how the brain processes visual information and how AI can bridge the gap between neural signals and perception.
Application to Clinical Neuroscience
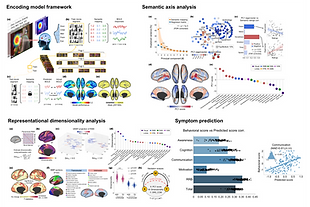

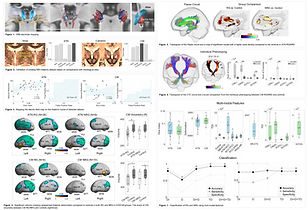
Whole-brain mapping of atypical semantic representation in autism
Transdiagnostic mapping of fronto-straital circuits in autism and ADHD
Connectome-based modeling of DBS effects in epilepsy

Jongeun Lee 이종은

Hanbyul Cho 조한별

Yejin An 안예진
We perceive the world not as raw sensory inputs but through concepts shaped by prior knowledge, facilitating efficient categorization. Individuals with autism spectrum disorder (ASD), however, tend to rely more on sensory details, leading to atypical conceptualization and restricted behaviors. Previous research on category learning in ASD is limited by artificial experimental settings. Our project uses naturalistic movie-watching fMRI with semantic encoding models to explore differences in conceptual systems, semantic processing, and developmental trajectories in ASD. Ultimately, we aim to link perceptual anomalies to conceptual differences and symptom severity in ASD.
My research aims to analyze and elucidate the relationship between neurobiological abnormalities and behavioral symptoms in neurodevelopmental disorders using functional connectivity and circuit information between the cortex and subcortex through a transdiagnostic approach. In particular, my research focuses on the comorbidity of ASD and ADHD diagnostic groups, as well as abnormalities in their corticostriatal circuits and executive function. This research project is named "Transdiagnostic Corticostriatal Circuit Mapping and Behavioral Modeling in ASD and ADHD."
My current focus is on deep brain stimulation (DBS) targeting the thalamic nuclei for epilepsy patients. To assess the effectiveness of DBS, I utilize a combination of structural, diffusion, and functional MRIs. Additionally, through computational modeling, I investigate the underlying mechanisms by which DBS interacts with the brain to improve clinical outcomes for patients.

Lesion synthesis using generative AI for data augmentation
